Review: 1
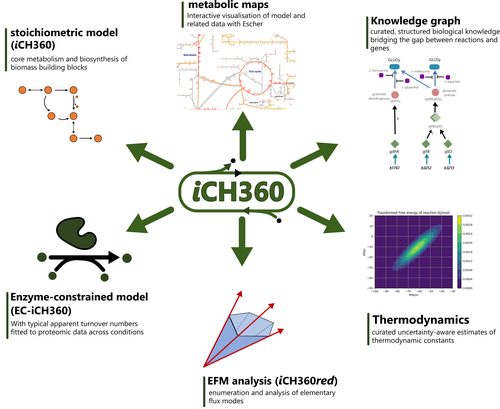
A compact model of Escherichia coli core and biosynthetic metabolism
‘Goldilocks’-size extensively annotated model for Escherichia coli metabolism
Recommended by Meike Wortel based on reviews by Daan de Groot, Benjamin Luke Coltman and 1 anonymous reviewerMetabolism is the driving force of life and thereby plays a key role in understanding microbial functioning in monoculture and in ecosystems, from natural habitats to biotechnological applications, from microbiomes related to human health to food production. However, the complexity of metabolic networks poses a major challenge for understanding how they are shaped by evolution and how we can manipulate them. Therefore, many network-based methods have been developed to study metabolism.
With the vast increase of genomic data, genome-scale metabolic networks have become popular use. For these stoichiometric models, metabolic enzymes are predicted using genome data and subsequently algorithms are used to add reactions to construct a complete (biomass producing) metabolic network (e.g., Henry et al., 2010; Machado et al., 2018; see for an overview Mendoza et al., 2019). Many tools are being developed to make predictions with these models, usually variations of FBA (Orth et al., 2010), but also methods for community predictions (Scott Jr et al., 2023) and simulations in time and space (Bauer et al., 2017; Dukovski et al., 2021). The vast amount of sequencing data combined with the high-throughput possibilities of this method make it appealing, but there is a drawback: Namely that the automated construction of networks lacks accuracy and often curation is necessary before these models produce realistic and useful results. This is exemplified by recent studies of microbial metabolism that are better predicted by genome content only than by actual metabolic models (Gralka et al., 2023; Li et al., 2023).
On the other end are well-curated small-scale models of metabolic pathways. For those, knowledge of the enzymes of a pathway, their kinetic properties and (optionally) regulation by metabolites is incorporated in usually a differential equation model. Standard methods for systems of differential equations can be used to study steady-states and the dynamics of these models, which can lead to accurate predictions (Flamholz et al., 2013; van Heerden et al., 2014). However, the downside is that the methods are difficult to scale up and, for many enzymes, the detailed information necessary for these models is not available. Combined with computational challenges, these models are limited to specific pathways and cannot be used for whole cells, nor even communities. Therefore, there is still a need for both methods and models to make accurate predictions on a scale beyond single pathways.
Corrao et al. (2025) aim for an intermediate size model that is both accurate and predictive, does not need an extensive set of enzyme parameters, but also encompasses most of the cell’s metabolic pathways. As they phrase it: a model in the ‘Goldilocks’ zone. Curation can improve genome-scale models substantially but requires additional experimental data. However, as the authors show, even the well-curated model of Escherichia coli can sometimes show unrealistic metabolic flux patterns. A smaller model can be better curated and therefore more predictive, and more methods can be applied, as for example EFM based approaches. The authors show an extensive set of methodologies that can be applied to this model and yield interpretable results. Additionally, the model contains a wealth of standardized annotation that could set a standard for the field.
This is a first model of its kind, and it is not surprising that E. coli is used as its metabolism is very well-studied. However, this could set the basis for similar models for other well-studied organisms. Because the model is well-annotated and characterized, it is very suitable for testing new methods that make predictions with such an intermediate-sized model and that can later be extended for larger models. In the future, such models for different species could aid the creation of methods for studying and predicting metabolism in communities, for which there is a large need for applications (e.g. bioremediation and human health).
The different layers of annotation and the available code with clear documentation make this model an ideal resource as teaching material as well. Methods can be explained on this model, which can still be visualized and interpreted because of its reduced size, while it is large enough to show the differences between methods.
Although it might be too much to expect models of this type for all species, the different layers of annotation can be used to inspire better annotation of genome-scale models and enhance their accuracy and predictability. Thus, this paper sets a standard that could benefit research on metabolic pathways from individual strains to natural communities to communities for biotechnology, bioremediation and human health.
References
Bauer, E., Zimmermann, J., Baldini, F., Thiele, I., Kaleta, C., 2017. BacArena: Individual-based metabolic modeling of heterogeneous microbes in complex communities. PLOS Comput. Biol. 13, e1005544. https://doi.org/10.1371/journal.pcbi.1005544
Corrao, M., He, H., Liebermeister, W., Noor, E., Bar-Even, A., 2025. A compact model of Escherichia coli core and biosynthetic metabolism. https://doi.org/10.48550/arXiv.2406.16596
Dukovski, I., Bajić, D., Chacón, J.M., Quintin, M., Vila, J.C.C., Sulheim, S., Pacheco, A.R., Bernstein, D.B., Riehl, W.J., Korolev, K.S., Sanchez, A., Harcombe, W.R., Segrè, D., 2021. A metabolic modeling platform for the computation of microbial ecosystems in time and space (COMETS). Nat. Protoc. 16, 5030–5082. https://doi.org/10.1038/s41596-021-00593-3
Flamholz, A., Noor, E., Bar-Even, A., Liebermeister, W., Milo, R., 2013. Glycolytic strategy as a tradeoff between energy yield and protein cost. Proc. Natl. Acad. Sci. 110, 10039–10044. https://doi.org/10.1073/pnas.1215283110
Gralka, M., Pollak, S., Cordero, O.X., 2023. Genome content predicts the carbon catabolic preferences of heterotrophic bacteria. Nat. Microbiol. 8, 1799–1808. https://doi.org/10.1038/s41564-023-01458-z
Henry, C.S., DeJongh, M., Best, A.A., Frybarger, P.M., Linsay, B., Stevens, R.L., 2010. High-throughput generation, optimization and analysis of genome-scale metabolic models. Nat. Biotechnol. 28, 977–982. https://doi.org/10.1038/nbt.1672
Li, Z., Selim, A., Kuehn, S., 2023. Statistical prediction of microbial metabolic traits from genomes. PLOS Comput. Biol. 19, e1011705. https://doi.org/10.1371/journal.pcbi.1011705
Machado, D., Andrejev, S., Tramontano, M., Patil, K.R., 2018. Fast automated reconstruction of genome-scale metabolic models for microbial species and communities. Nucleic Acids Res. 46, 7542–7553. https://doi.org/10.1093/nar/gky537
Mendoza, S.N., Olivier, B.G., Molenaar, D., Teusink, B., 2019. A systematic assessment of current genome-scale metabolic reconstruction tools. Genome Biol. 20, 158. https://doi.org/10.1186/s13059-019-1769-1
Orth, J.D., Thiele, I., Palsson, B.Ø., 2010. What is flux balance analysis? Nat. Biotechnol. 28, 245–248. https://doi.org/10.1038/nbt.1614
Scott Jr, W.T., Benito-Vaquerizo, S., Zimmermann, J., Bajić, D., Heinken, A., Suarez-Diez, M., Schaap, P.J., 2023. A structured evaluation of genome-scale constraint-based modeling tools for microbial consortia. PLOS Comput. Biol. 19, e1011363. https://doi.org/10.1371/journal.pcbi.1011363
van Heerden, J.H., Wortel, M.T., Bruggeman, F.J., Heijnen, J.J., Bollen, Y.J.M., Planqué, R., Hulshof, J., O’Toole, T.G., Wahl, S.A., Teusink, B., 2014. Lost in Transition: Start-Up of Glycolysis Yields Subpopulations of Nongrowing Cells. Science 343, 1245114. https://doi.org/10.1126/science.1245114