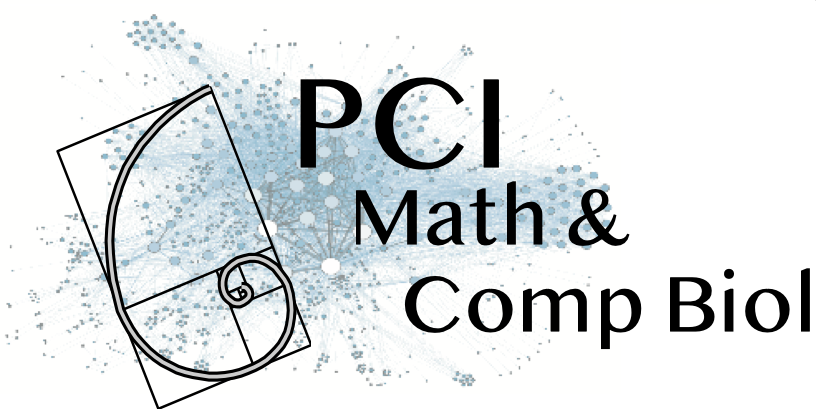
Importance of age structure on modeling COVID-19 epidemiological dynamics
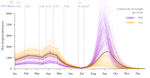
Non-Markovian modelling highlights the importance of age structure on Covid-19 epidemiological dynamics
Abstract
Recommendation: posted 30 January 2022, validated 04 February 2022
Liao, C. (2022) Importance of age structure on modeling COVID-19 epidemiological dynamics. Peer Community in Mathematical and Computational Biology, 100008. https://doi.org/10.24072/pci.mcb.100008
Recommendation
COVID-19 spread around the globe in early 2020 and has deeply changed our everyday life [1]. Mathematical models allow us to estimate R0 (basic reproduction number), understand the progression of viral infection, explore the impacts of quarantine on the epidemic, and most importantly, predict the future outbreak [2]. The most classical model is SIR, which describes time evolution of three variables, i.e., number of susceptible people (S), number of people infected (I), and number of people who have recovered (R), based on their transition rates [3]. Despite the simplicity, SIR model produces several general predictions that have important implications for public health [3].
SIR model includes three populations with distinct labels and is thus compartmentalized. Extra compartments can be added to describe additional states of populations, for example, people exposed to the virus but not yet infectious. However, a model with more compartments, though more realistic, is also more difficult to parameterize and analyze. The study by Reyné et al. [4] proposed an alternative formalism based on PDE (partial differential equation), which allows modeling different biological scenarios without the need of adding additional compartments. As illustrated, the authors modeled hospital admission dynamics in a vaccinated population only with 8 general compartments.
The main conclusion of this study is that the vaccination level till 2021 summer was insufficient to prevent a new epidemic in France. Additionally, the authors used alternative data sources to estimate the age-structured contact patterns. By sensitivity analysis on a daily basis, they found that the 9 parameters in the age-structured contact matrix are most variable and thus shape Covid19 pandemic dynamics. This result highlights the importance of incorporating age structure of the host population in modeling infectious diseases. However, a relevant potential limitation is that the contact matrix was assumed to be constant throughout the simulations. To account for time dependence of the contact matrix, social and behavioral factors need to be integrated [5].
References
[1] Hu B, Guo H, Zhou P, Shi Z-L (2021) Characteristics of SARS-CoV-2 and COVID-19. Nature Reviews Microbiology, 19, 141–154. https://doi.org/10.1038/s41579-020-00459-7
[2] Jinxing G, Yongyue W, Yang Z, Feng C (2020) Modeling the transmission dynamics of COVID-19 epidemic: a systematic review. The Journal of Biomedical Research, 34, 422–430. https://doi.org/10.7555/JBR.34.20200119
[3] Tolles J, Luong T (2020) Modeling Epidemics With Compartmental Models. JAMA, 323, 2515–2516. https://doi.org/10.1001/jama.2020.8420
[4] Reyné B, Richard Q, Noûs C, Selinger C, Sofonea MT, Djidjou-Demasse R, Alizon S (2022) Non-Markovian modelling highlights the importance of age structure on Covid-19 epidemiological dynamics. medRxiv, 2021.09.30.21264339, ver. 3 peer-reviewed and recommended by Peer Community in Mathematical and Computational Biology. https://doi.org/10.1101/2021.09.30.21264339
[5] Bedson J, Skrip LA, Pedi D, Abramowitz S, Carter S, Jalloh MF, Funk S, Gobat N, Giles-Vernick T, Chowell G, de Almeida JR, Elessawi R, Scarpino SV, Hammond RA, Briand S, Epstein JM, Hébert-Dufresne L, Althouse BM (2021) A review and agenda for integrated disease models including social and behavioural factors. Nature Human Behaviour, 5, 834–846 https://doi.org/10.1038/s41562-021-01136-2
The recommender in charge of the evaluation of the article and the reviewers declared that they have no conflict of interest (as defined in the code of conduct of PCI) with the authors or with the content of the article. The authors declared that they comply with the PCI rule of having no financial conflicts of interest in relation to the content of the article.
Evaluation round #1
DOI or URL of the preprint: https://doi.org/10.1101/2021.09.30.21264339
Version of the preprint: 1
Author's Reply, 20 Jan 2022
Decision by Chen Liao, posted 30 Dec 2021
Dear Authors,
We have received three reviews of your manuscript, two of which are very thoughful and detailed. All three reviwers are positive and appreciate the mathematical approaches proposed in your study. Given that this is a solid work with rigorous methodology and well-structured texts, we are happy to recommend your article after some minor revisions according to the review recommendations. In particular, I would encourage the authors to improve the following two aspects: (1) literature review of other PDE approaches and (2) codes/documentation of the software package.
Please submit your revised manuscript within one month and let us know if you anticipate any delay.
When you are ready to resubmit, please provide a detailed list of your responses to all review comments and a desription of the changes you have made in the manuscript. I would have appreciated if two versions of the revised manuscript are provided: one clean version and the other denoting where the text has been changed (highlighted or in track-change).
We hope that our recommendation process has been constructive so far. Please don't hesitate to contact us if you have any questions or comments.
Sincerely,
Chen Liao
Reviewed by anonymous reviewer 1, 02 Dec 2021
Reviewed by Facundo Muñoz, 16 Nov 2021
# Review of "The importance of the population age-structure: insights from Covid-19 dynamics model structured by age, time since infection and acquired immunity"
https://doi.org/10.1101/2021.09.30.21264339
Facundo Muñoz, November 2021.
## General considerations
Main goal: Demonstrate the adaptation and extension of a recently published (by some of the same authors) approach which overcomes the Markovian hypothesis (lack of memory) of classical compartmental epidemiological models, with the objective of understanding the interplay between vaccination rates and age-structure in the Covid-19 pandemic in France.
Method: The methodology generalises the classical methodology based on Ordinary Differential Equations (ODEs) with respect to __time__, with Partial Differential Equations (PDEs) with respect to the __age__ and to the __time since infection__, in addition to __time__.
The authors deploy a specific compartment for vaccinated population and explore the use of alternative data sources to inform the age-structured contact patterns.
I think the title and the introduction could be improved to better identify the main topic of the manuscript.
The title focus on the scientific questions about some mechanisms at play in the French Covid-19 pandemic ("the importance of the population age-structure"), without any mention to the methodology.
By contrast, the abstract and introduction focus on the methodology, presenting the lack-of-memory issue as the knowledge gap to be addressed: "... we introduce an alternate formalism relying on partial differential equations..." (l. 35).
In my first read of the manuscript, I thought that the main topic was the methodology and the title was highlighting an application. It took me a second, more careful read to understand that the methodology had been introduced previously in Richard et al. (2021) and the present paper demonstrated how to tailor it to address different questions. But this is not clearly conveyed by neither the title nor the introduction (or the abstract).
## Introduction
The section is very well structured, first stating the context and quickly identifying the knowledge gap. Namely, the need to model memory effects.
It then explains the limits of two prior alternative approaches and makes a good case by arguing that multiplying compartments do not scale well and models quickly become very difficult to parameterise and interpret.
However, the first method is dismissed as a "workaround" which "artificially" increases the number of compartments.
I think these are inappropriate dismissive qualifiers. Every model could be ultimately considered as _artificial_ and used to _work around_ reality. The question is how _useful_ they are.
More specific statements about the relative merits would be much more informative. For instance, the authors could rather argue that modelling heterogeneities by age __continuously__ is more _parsimonious_ than introducing __artificial__ boundaries between age groups. This formulation explicitly specifies what exactly is being considered _artificial_, by contrast to the current proposal.
I would have appreciated further introductory references to epidemiological modelling with PDEs. The only reference is Richard et al. (2021), which in turn says that it is a "less common and much more challenging" approach, without further references.
## Materials and methods
The presentation of the model is condensed, but well structured, rigorous and sufficiently detailed. Especially given that the main ideas were presented previously in some more detail.
I have only missed one or two sentences to discuss the recovery rate $\gamma^{mv}(a, i)$ from compartment $I^{mv}_{aik}$, about line 70, where the need for this compartment is introduced. In particular, justifying the choice for recovered individuals returning back to the compartment $V_{ak}$ rather than $R_{aj}$. Stating explicitly that, in so doing, the time since vaccination $k$ is preserved, and possibly other consequences of the choice.
l. 75: « ... the number of [+newly] severely infected individuals of age $a$ at time $t$ [-is][+are] given by the boundary condition[+s] »
In point 6 of Assumption S1, I think it is missing the case $l \in d$, or is there a reason for leaving it out?
I must confess that I could not quite follow the demonstration of the well-posedness of the system in appendix A.2, nor the derivation of the basic reproduction number in appendix A.3. It's been a long time since I last revisited Banach spaces, and I am not familiar with the utilised methods and results. Nevertheless, both sections provide enough references and pointers for interested readers.
## Results
All the data and code were appropriately available for reproducing the results.
Providing cached intermediate results which are lengthy to compute is very much appreciated.
However, the documentation and comments are not sufficiently detailed.
For instance, the first script (`1_fit_vaccionation.R`) performs a calculation in parallel, which seems computationally demanding (I stopped it after a few minutes). It stores the results into an object called `results`.
Coincidentally, there is a cached data file named `results.RData` which, judging by the name, seems to correspond with said computation.
Yet there is no comment or indication confirming this, and loading such data file brings in a number of objects, none of them called _results_.
It takes some more investigation to figure out that `results.RData` is created by the 5th script, and used in the 7th. So, it seems related to something else.
Next, the second script warns from the beginning that it takes a few hours to run. Yet, it does not provide any pointer to the generated object (called `best` in the script), for which there is no cached results.
I don't pretend to be overly critic. It is apparent that the authors put some effort in cleaning up and commenting their code, and I truly appreciate it.
Still, making code available and __accessible__ to other people is difficult and takes a lot of time. Sometimes as much as producing the code itself.
The R package `modelvacc` is a wrapper around a set of C++ functions that implement the model equations and procedures.
However, its complete lack of documentation (code comments, help pages) and tests somewhat hampers its reliability and re-usability by other researchers. I believe that this package is of considerable scientific value as a companion to the paper and can be instrumental in the adoption and improvement of the approach proposed by the authors. As such, it should be subject to the same high standards as the manuscript itself.
In summary, I would encourage the editors and the authors to improve the code a bit before, or after, publication.
## Discussion
The discussion is well structured, placing the results in context, and stating the relevant scientific conclusions given the strengths and limitations of the approach.
https://doi.org/10.24072/pci.mcb.100111.rev12
Reviewed by Kevin Bonham, 20 Dec 2021
Reyne et. al. - The importance of the population age-structure: insights from Covid-19dynamics model structured by age, time since infection and acquired immunity
Review
In The importance of the population age-structure: insights from Covid-19 dynamics model structured by age, time since infection and acquired immunity, Reyné and colleagues present a SIR model based on partial differential equations (PDE) as opposed to the typical ODE-based models. The authors state that this provides the ability to more faithfully capture the time that individuals spend within model compartments (memory) without the need to artifically inflate the number of compartments modeled. This has the advantage of increasing interpretability and flexibility of the model at the cost of more up-front effort at parameterization.
Unfortunately, I fear I lack the mathematical expertise to comment directly on the construction of the model and on its outputs. I will instead focus on the clarity of the writing and on the software, in the hopes that this will be useful.
Writing
The authors do an admirable job explaining the construction of their PDE model, including how individual terms relate to real-world scenarios and the source of values for initial parameterization. Though I am not able to readily follow the math, the descriptions in the text are clear and sensible. Figure 1 provides a useful reference for the modeled compartments, and the pathways between them.
Many of the limitations that I perceived are mentioned in the main text or in the discussion, and are adequately explained. One exception here is regarding the waning of immunity after vaccination (lines 104-105).
Regarding the modelling of vaccine efficacy, for simplicity, we neglect immune waning, i.e. the decrease of immunity with time
The time-dependent changes in vaccine effectiveness strike me as a major source of uncertainty in this pandemic, and something for which models of this sort are well-suited to address (as claimed by the authors on line 34 as one motivation for this approach). In other portions of the manuscript, the authors imply that they are modeling this waning (eg ln 67 and ln 286). Perhaps it is clear from the equations, but I find myself unclear on whether this is actually accounted for or not.
Software
The authors make their software (written in C++ and R) available via an institutional gitlab repository. I was able to download a tarball of this code and follow the instructions to install dependencies on my laptop (Ubuntu xenial, R v4.0.1) Though (as mentioned in the README) many of the scripts take a long time to run, intermediate results are helpfully provided, and all of the code that I tried ran without errors until I interrupted it. The R portions of the code contain many helpful comments.
There are a few places where values that should perhaps be determined programmatically are hard-coded (eg here), and it might be nice if the parameters described in the paper could be found in a single configuration file (or something) rather than sprinkled throughout the scripts, as this would make it easier to tweak the assumptions of the model to see their effects, but these are very minor gripes.
I find it quite admirable that code is provided in a runnable state for review. A few additional steps could make this code availability even stronger (though I hesitate to demand any of these steps as necessary).
- Register / archive the code via an independent institutional repository such as zenodo.org or osf.io. Especially one that provides a digital object identifier (DOI). As it stands, there is no guarantee that this code won't disappear tomorrow.
- Provide additional instructions for installing specific versions of packages. The provided session_info.txt file is a great start - using the renv package allowed me to reproduce the environment, at least as regards R dependencies. Additional information about C++ versions and compilation would also be welcome.
- Provide some kind of indication within the scripts (just comments would be fine) which portions of the code take approximately what amount of time. I would have like to try to run the code that only takes minutes or hours so that I could inspect the output, but without knowing which parts might take days (or be infeasible on my laptop), this isn't practically possible.
- Descriptions in the code that reference specific parts of the paper. Especially given my difficulty with understanding the math, being able to link the code directly to descriptions in the paper would be immensely helpful.
The commit history on the publicly available project looks like it starts when the project was basically complete. Many people are uncomfortable sharing in-progress code (it's possible that version tracking was not even done earlier), and I don't think anything different is expected, which is why I'm not including it in my list of suggestions. But it's a shame.
Results
The results, so far as I understand them, are impressive and on the whole, clearly presented. I am a bit unclear about figure 2 - in particular, I wonder if it would make more sense to split the ages into more plausible units, rather than just 10 year increments. For example, infants and toddlers are likely to be more dissimilar from school age kids than eg 9 vs 11 year olds. One might also consider breaking up based on availability of vaccine (eg the youngest kids still can't get vaccinated).
For final publication, it might be nice to extend figures 5 and 6 with the most recent available data, as it currently ends in August. I don't know how feasible this is given the run-time of the code. Any further deviations from reality would not necessarily change the utility of this paper, but could be interesting fodder for discussion.
https://doi.org/10.24072/pci.mcb.100111.rev13