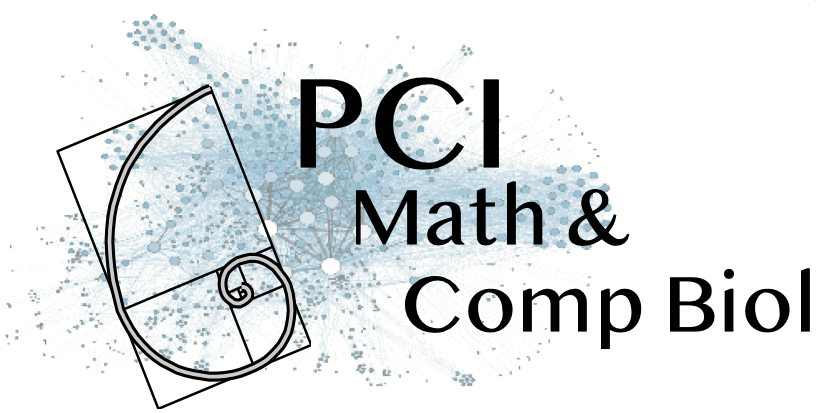
A computational method to identify key players in metabolic rewiring
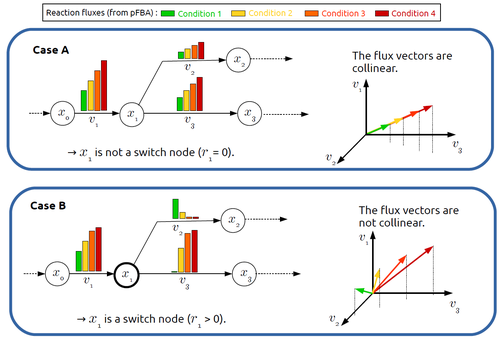
In silico identification of switching nodes in metabolic networks
Abstract
Recommendation: posted 24 September 2024, validated 27 September 2024
Chaouiya, C. (2024) A computational method to identify key players in metabolic rewiring. Peer Community in Mathematical and Computational Biology, 100193. 10.24072/pci.mcb.100193
Recommendation
Significant progress has been made in developing computational methods to tackle the analysis of the numerous (genome-wide scale) metabolic networks that have been documented for a wide range of species. Understanding the behaviours of these complex reaction networks is crucial in various domains such as biotechnology and medicine.
Metabolic rewiring is essential as it enables cells to adapt their metabolism to changing environmental conditions. Identifying the metabolites around which metabolic rewiring occurs is certainly useful in the case of metabolic engineering, which relies on metabolic rewiring to transform micro-organisms into cellular factories [1], as well as in other contexts.
This paper by F. Mairet [2] introduces a method to disclose these metabolites, named switch nodes, relying on the analysis of the flux distributions for different input conditions. Basically, considering fluxes for different inputs, which can be computed using e.g. Parsimonious Flux Balance Analysis (pFBA), the proposed method consists in identifying metabolites involved in reactions whose different flux vectors are not collinear. The approach is supported by four case studies, considering core and genome-scale metabolic networks of Escherichia coli, Saccharomyces cerevisiae and the diatom Phaeodactylum tricornutum.
Whilst identified switch nodes may be biased because computed flux vectors satisfying given objectives are not necessarily unique, the proposed method has still a relevant predictive potential, complementing the current array of computational methods to study metabolism.
References
[1] Tao Yu, Yasaman Dabirian, Quanli Liu, Verena Siewers, Jens Nielsen (2019) Strategies and challenges for metabolic rewiring. Current Opinion in Systems Biology, Vol 15, pp 30-38. https://doi.org/10.1016/j.coisb.2019.03.004.
[2] Francis Mairet (2024) In silico identification of switching nodes in metabolic networks. bioRxiv, ver.3 peer-reviewed and recommended by PCI Math Comp Biol https://doi.org/10.1101/2023.05.17.541195
The recommender in charge of the evaluation of the article and the reviewers declared that they have no conflict of interest (as defined in the code of conduct of PCI) with the authors or with the content of the article. The authors declared that they comply with the PCI rule of having no financial conflicts of interest in relation to the content of the article.
LEFE Project HétéroMixo
Reviewed by anonymous reviewer 2, 05 Sep 2024
While some questions remain unresolved, the author has satisfactorily addressed most of my concerns. I have no additional comments.
Evaluation round #2
DOI or URL of the preprint: https://doi.org/10.1101/2023.05.17.541195
Version of the preprint: 2
Author's Reply, 24 Jul 2024
Decision by Claudine Chaouiya
, posted 05 Jul 2024, validated 05 Jul 2024
Dear author,
We have now received the reviews, which both aknowledge a significant improvement of the manuscript but also point to some clarifications that need to be consider.
I thus suggest a minor revision, carefully addressing reviewers' comments.
Best regards
Claudine Chaouiya
Reviewed by anonymous reviewer 1, 05 Jul 2024
I would like to thank the author for the changes made in manuscript, which is now clearer and sounder.
Analyses are reproducible, all data and scripts are available on Github.
My remaining comments are minor:
- I am still doubful about the use of "(somehow overlooked)" phrase in the abstract. I believe "suggesting the importance" is well enough and less subjective.
- P2 l53: "we" --> "I" for consistency with the rest of the text?
- Fig4: consider adding in the graphical legend the signification of the node width (switch node score)
- Fig6: what is plotted is not clear: in x the dot is the average and the std is plotted as vertical bars?
- In the discussion (L197 p10) could the discrepencies occur simply because FBA does not account for transcriptional regulation, therefore some switch metabolites could be false positives? Could constraining fluxes while taking additional data into account (transcriptomics), or maybe using alternatives to FBA (eg RBA? https://doi.org/10.1042/BST20160436) to compute the fluxes highlight more biologically relevant switches?
Reviewed by anonymous reviewer 2, 02 Jul 2024
The author has significantly improved the manuscript's flow and clarity by providing additional explanations, details, and comparisons to other existing methods in this area.
Regarding my previous remark about the possible bias in identifying switches due to variability in flux solutions, the newly introduced section, “Robustness to Flux Sampling,” is a welcome addition and clearly demonstrates why alternative flux solutions should be analyzed. The author observed that multiple metabolites, not identified in the original study as switches, became switches when studying alternative solutions, and vice versa.
This observation raises questions about the reliability of identified metabolic switches in the studies preceding the introduced section. The author’s statement on page 8, lines 169-170, “Exploring the flux space is therefore useful to confirm the identification of switch points, but it also leads to artificial switch points,” is misleading. Exploring the flux space allows us to consider all possible outcomes given the experimentally observed data and network topology, whereas considering only one arbitrary flux solution provides just one snapshot. Therefore, the claim that sampling leads to “artificial switch points” is not necessarily accurate.
Similarly, on page 10, lines 201-202, the statement “false positives may be generated” follows the same reasoning. One may ask what the ground truth is on which the author bases their conclusion about the false positivity of these outcomes.
A common approach to addressing such bias in this line of research is to explore the flux solution space with a statistically significant number of samples, analyze the obtained distributions, and draw conclusions based on chosen statistical criteria. This method would allow the author to provide a probability measure of identified switches. I recommend such an approach for the presented studies, as the current conclusions may be drawn from a flux solution that is an outlier (or on the tails) in the distribution of flux solutions.
Additionally, the number of samples (1000) in the provided robustness sampling study is insufficient for statistical significance in the case of large-scale metabolic networks.
Minor Corrections:
Page 4, line 108: “shows” should be changed to “show.”
Page 8, line 159: “vey” should be corrected to “very.”
Evaluation round #1
DOI or URL of the preprint: https://doi.org/10.1101/2023.05.17.541195
Version of the preprint: 1
Author's Reply, 07 Jun 2024
Decision by Claudine Chaouiya
, posted 23 Jul 2023, validated 24 Jul 2023
Dear author,
We have now received the reports of two independent peer reviewers. Both raised substantial concerns
about the proposed method and its validity. They provide suggestions to improve the manuscript.
Therefore, I consider that I cannot recommend the preprint in its current version, but I would be willing to review a much-revised version that addresses reviewers concerns.
Best regards,
Claudine Chaouiya
Reviewed by anonymous reviewer 1, 10 Jul 2023
In this paper, the author presents a constraint-based approach for the identification of key metabolites in metabolic networks. Such molecules, denoted as "switch metabolites" are identified using parsimonious flux balance analysis by comparing the flux distributions in the network obtained with multiple nutrient inputs. Fluxes are reoriented depending around these metabolites depending on the environmental conditions.
The manuscript is overall well-written.
The approach proposed by the author is interesting but the manuscript would be more convincing should more context, precisions and justifications be added. There is no convincing validation of the results provided by the approach. Sections are quite short, sometimes very short, more details could be added without making the manuscript excessively long. To my opinion, the conclusions on the relevance of the approach are not sufficiently supported by the results, although I believe that the work has a potential.
Introduction:
The introduction is a bit short for non-experts and could better motivate the research question.
Previous studies and or approaches are listed as references but they are not discussed, even briefly, making it complicated for a non-expert reader to grasp the context of the current study.
- Two consecutive sentences starting with "More and more"
- A description of FBA that does not mention its main characteristics
- Paragraph 2: no reference on the complexity of the analysis of large newtorks, or the fact that identification of key metabolites is a major concern: how will an answer to this question help tackle the complexity of metabolic networks?
- Existing methods for identifying key metabolites and the vision of the author on switch points could also be summarised in the text rather than simply being referred to.
Results:
- E. coli simulations
* The names of the metabolites in Fig 2C are hardly visible.
* "This is in line with what we could expect..." L72. Any reference?
- S. cerevisiae simulations
* The three sizes of the pvalues/scores are hard to decipher in Fig3. Consider adding color?
* The text is brief, does not mention the number of switch metabolites wrt to reporters for instance.
* Few metabolites are common between reporters and switch metabolites in Fig 3.
* L89: "several reporter metabolites appears in the top of the switch metabolite list (see SI), even if they do not appear in Fig. 3": why do they not appear in Fig3? Please mention the contents of each SI (in the github repository) you refer to, somewhere in the text (after methods?)
- A rank of switch metabolites is mentioned L91. Are all metabolites of the network switches but with different scores, or are a subset of the nodes switches based on a threshold? Out of the total of 2241 nodes, many of them have a score of 0, so shouldn't be described as switches I guess.
- P. tricornatum simulations
* is the role of erythrose-4-phosphate validated in the literature? There are no references in the text. Also this is presented in the abstract as a highlight but I do not see validation of the role of the metabolite. Please rephrase the sentence of the abstract with the mention "highlighting the (somehow overlooked) importance" if there is no validation.
Discussion:
- "The methods gives sound results" L126. The validation of the relevance of the approach is only addressed in the PTM analysis
- The results on the reporter analysis on the yeast are presented positively: "One of the closer definition is that of reporter metabolites [18], and the results on a case study have shown some similarities (see the case study with S. cerevisiae). The main advantage of ISIS is that it does not require experimental data.". But one could see the glass half empty: a lot of the reporter metabolites were not identified as switches.
- A justification for the absence of comparison with other methods is the difference in the definition of a key metabolite (L130): why would the definition advocated for in the manuscript be (more) biologically relevant?
Methods:
Additional precisions should be added to facilitate the understanding what has been performed in the work.
- A general comment on the section describing ISIS principle is that it lacks justifications on the mathematical choices, making it hard to follow for a non expert. This is the core of the approach presented in the manuscript and the underlying reasons of the modelling choices that were done are not provided. Here, pFBA has been used, which is understandable because it provides a unique optimal solution. Yet, the author mentions several times that other approaches could be used: would it have an impact on the results or are the switch metabolites robust to the optimisation choice?
- Is there a notion of threshold that is used to determine whether the metabolite is a switch or not, according to its score $r_i$? How/why were the thresholds of 0.1, 0.3, 0.5 chosen in the results?
- The definitions of FBA and pFBA could be more precisely described.
* The FBA problem has two types of constraints: the steady state assumption (SSA), and the inequality constraints of flux bounds. The latter is not presented as a main constraint of the problem at the same level as the SSA. In addition, the objective function in FBA does not have to be a maximisation. Theoretically, it can also be a minimisation.
* "Then, we determine the solution with the minimum overall flux that have (almost) the same objective value" L169 misses precision as well. The sum of fluxes is minimised. "Almost" in imprecise.
- L174: Could you give more details on the normalisation? By the sum of fluxes in the model?
- For all simulations, I assume the biomass reaction was chosen as an objective function? This is not mentioned for any of the case studies.
- S. cerevisiae simulations.
* L195 "The switch metabolites are computed two by two". Could you precise the meaning (also used in results)?
* L194 mention some details about the remaining compounds of the medium?
* L196: give access to the list of currency metabolites used, or maybe the raw results? Why removing them as you state that they are mentioned as switch metabolites and seem important in E. coli core?
- E. coli simulations
- L200 what do you mean "with all the conditions at once"?
- P. tricornatum simulations
- Are the flux simulations obtained with pFBA as well? Context on the study from which the data was taken is missing.
- There is no section on statistics but tests are used in results (eg L84)
Implementation:
The implementation of the tool in Python 2.7 is a bit surprising as the support for this version of Python has ended 3 years ago. For ensuring the usage of the method by the community, the author could consider upgrading the code to a more recent version. First tests on the notebooks provided seem to show compatibility.
It is not clear in the paper whether the implementation of the method is a main contribution of the work or not. At the moment, based on the repository, it would seem it is not: no installation procedure (library dependencies), no information on the versions used (eg cobrapy L182, Escher L192)... If the implementation is a contribution, the author might consider building a package out of the code, and using the notebooks as a demonstration support.
A more general remark on the writing, that is maybe personal. The author uses a lot of parentheses to give additional information in the text, sometimes several occurrences in a single sentence (L175,176), or to mention rather important information (L92). I personally find that it disrupts the reading and makes sentences harder to understand.
Reviewed by anonymous reviewer 2, 07 Jul 2023
The author proposes a method for determining the metabolic hubs around which metabolism rewiring occurs under different physiological conditions. The technique identifies the switching nodes by testing the algebraic collinearity of flux vectors corresponding to the reactions involving the studied metabolites. The author showcases the method through four case studies. The text is well-structured and relatively clearly written. However, many details are missing, and it would be challenging to replicate the study results.
Whereas the author claims the generality of the approach, i.e., that it can use any set of flux solutions from different conditions, pFBA is used in all studies with the assumption that pFBA is giving a unique solution – which allows the author to use 1 “representative” flux vector per condition. However, except for specific and relatively small metabolic networks, pFBA will not give a unique solution, i.e., it will also allow multiple flux vectors satisfying both the objective (e.g., optimal growth) and the minimal sum of the fluxes. In my experience, it will even enable some reactions to operate in both forward and reverse direction (i.e., specific fluxes could take both positive and negative values). And this will give a significant bias in the provided results. In general, variability in the fluxes within each condition case will also affect the proposed measure. This should be studied and clarified.
Since the proposed method identifies the key metabolites by studying fluxes and their dependencies, it reminds of the flux coupling (FC) methods. Can one use FC methods or inspire from them in the proposed approach? The author should discuss how this is different/ how it improves over the flux coupling approaches.