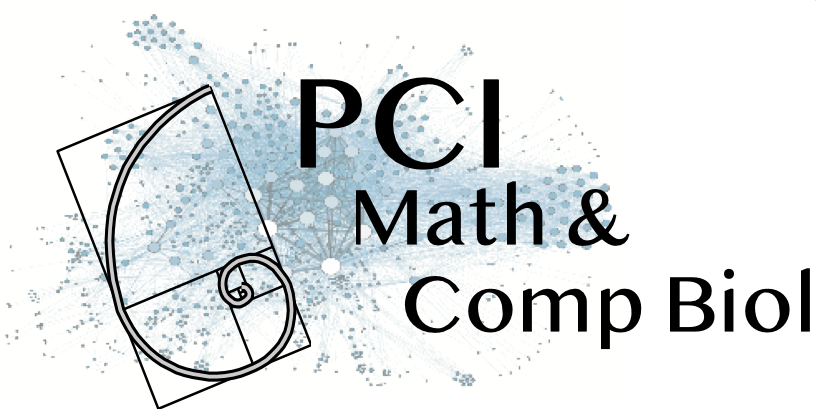
Optimizing antibiotic use: How much should we favor drugs with lower resistance mutation rates?
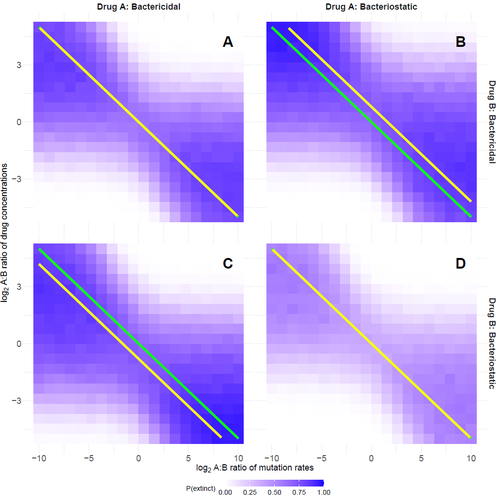
Optimal antimicrobial dosing combinations when drug-resistance mutation rates differ
Abstract
Recommendation: posted 30 March 2025, validated 30 March 2025
Lambert, A. (2025) Optimizing antibiotic use: How much should we favor drugs with lower resistance mutation rates?. Peer Community in Mathematical and Computational Biology, 100312. 10.24072/pci.mcb.100312
Recommendation
In the hereby recommended paper [1], Delaney, Letten and Engelstädter study the appearance of antibiotic resistance(s) in a bacterial population subject to a combination of two antibiotics \( A \) and \( B \), in concentrations \( C_A \) and \( C_B \) respectively. Their goal was to find optimal values of \( C_A \) and \( C_B \) that minimize the risk of evolutionary rescue, under the unusual assumption that resistance mutations to either antibiotic are not equally likely.
The authors introduce a stochastic model assuming that the susceptible population grows like a supercritical birth-death process which becomes subcritical in the presence of antibiotics (and exactly critical for a certain concentration c of a single antibiotic): the effect of each antibiotic is either to reduce division rate (bacteriostatic drug) or to enhance death rate (bacteriocidal drug) by a factor which has a sigmoid functional dependence on antibiotic concentration.
Now at each division, a resistance mutation can arise, with probability \( \mu_A \) for a resistance to antibiotic \( A \) and with probability \( \mu_B \) for a resistance to antibiotic \( B \).
The goal of the paper is to find the optimal ratio of drug concentrations \( C_A \) and \( C_B \) when these are subject to a constrain \( C_A + C_B = c \), depending on the ratio of mutation rates. Assuming total resistance and no cross resistance, the authors show that the optimal concentrations are given by \( C_A = c/(1+\sqrt{\mu_A/\mu_B }) \) and \( C_B = c/(1+\sqrt{\mu_B/\mu_A}) \), which leads to the beautiful result that the optimal ratio \( C_A/C_B \) is equal to the square root of \( \mu_B/\mu_A \).
The authors have made a great job completing their initial submission by simulations of model extensions, relaxing assumptions like single antibiotic mode, absence of competition, absence of cost of resistance, sharp cutoff in toxicity… and comparing the results obtained by simulation to their mathematical result.
The paper is very clearly written and any reader interested in antibiotic resistance, stochastic modeling of bacterial populations and/or evolutionary rescue will enjoy reading it. Let me thank the authors for their patience and for their constant willingness to comply with the reviewers’ and recommender’s demands during the reviewing process.
Reference
Oscar Delaney, Andrew D. Letten, Jan Engelstaedter (2025) Optimal antimicrobial dosing combinations when drug-resistance mutation rates differ. bioRxiv, ver.3 peer-reviewed and recommended by PCI Mathematical and Computational Biology https://doi.org/10.1101/2024.05.04.592498
The recommender in charge of the evaluation of the article and the reviewers declared that they have no conflict of interest (as defined in the code of conduct of PCI) with the authors or with the content of the article. The authors declared that they comply with the PCI rule of having no financial conflicts of interest in relation to the content of the article.
The authors declare that they have received no specific funding for this study
Evaluation round #2
DOI or URL of the preprint: https://doi.org/10.1101/2024.05.04.592498
Version of the preprint: 2
Author's Reply, 20 Mar 2025
Decision by Amaury Lambert, posted 12 Feb 2025, validated 12 Feb 2025
Dear authors,
Thanks a lot for your revision and the care you brought to addressing all the comments by the reviewers and myself.
It took me much longer than expected to hand my decision, because each time I resumed reading the manuscript, it was slown down by some paragraph I had hard times grasping.
I am really close to recommending your nice paper, but I would appreciate your improving clarity by addressing the following (virtually all minor) points:
- line 97 “if antibiotic treatment is started early by the human host before pathogenic bacteria have reached the resource limits of their niche, our model could be approximately accurate.” I am not sure a model can be ‘accurate’ and I don’t think anything can be ‘approximately accurate’, please reformulate
- lines 85 and 100-101: can you specify that total resistance is equivalent to E_{M_A} (C_A) = E_{M_B} (C_B) = 0, which is basically equivalent to z_AA and z_BB infinite?
- lines 107-110, I understand your reasoning concerning the gates AND and OR, but I am not sure I am convinced by their translation in terms of birth and death rates. I think I can understand (in terms of competing exponential clocks) that it is equivalent to assume that the effects of drugs on death rate are summed and to say that ‘it is sufficient for either drug to cause its death’. But it does not seem equivalent (to me) to assume that the effects of drugs on division rate are multiplied and to say that ‘for a cell to divide both pathways impacted by the drugs must remain functional’.
- line 123 `to make evolving this stochastic system computationally feasible’: I’m not sure it is the right way to justify using tau-leaping – the system is really simple to simulate with a classical Doob-Gillespie algorithm. It’s more that you speed up simulations this way.
- line 136, z_{S,A} and z_{S,B} are specified, but nothing is said of the 4 other z’s. Also please give the units of \mu and \delta.
- lines 147-149, ‘The more important point for this simple theoretical model is to use parameter values that highlight biologically relevant phenomena, rather than using maximally likely parameter values’. I’m not sure to see what you mean. What are parameter values that ‘highlight biologically relevant phenomena’ if these parameter values are not ‘likely’? Do you mean parameter values do not need to be finely tuned, but only need to be in the right ballpark?
- line 171, you should explain the link between gambler’s ruin and probability of extinction (or just explain that (7) can be obtained directly by saying that extinction does occur iff at each division event, we don't have both a mutation event and the survival of this mutant)
- line 216, what do you mean by ‘directionally plausible’?
- Equation 14, I’m not sure the three dots (forming a triangle) are canonical to symbolize logical implication
- line 226, ‘if only A or B is used’, please add ‘that is, if C_A or C_B is zero’… (makes more clearly the link with the previous case when C_A=C_B)
- line 228, alternative formulation: ‘the survival probability of a mutant is more sensitive to an increase in concentration of the drug to which resistance mutations arise more frequently’
- Equation 15, I agree it is nice to have this relationship between C_A/C_B and \mu_A/\mu_B, however, this formulation does not highlight the dependence on C of the result, and suggests that there is a degree of freedom in the choice of C_A and C_B. It would be clearer to first state that at the optimal combination, we have C_A = C/(1+\sqrt{\mu_A/\mu_B }) and C_B = C\sqrt{\mu_A/\mu_B }/(1+\sqrt{\mu_A/\mu_B})
- The paragraph starting line 259 is somewhat obscure to me. We read that ‘As resistance becomes weaker (from the earlier unrealistic supposition of total resistance), the two resistant strains become less perfectly adapted to their respective drugs’, which means that E_{M_A} (C_A) and E_{M_B} (C_B) should be nonzero. It would be good to say that now z_{M_A, A} and z_{M_B, B} are finite (otherwise it is difficult to make the link with the case of total resistance). Also please change the caption of Figure 2 and S1, which only mentions z_{M_A, B} and z_{M_B, A}. We read that these two values are exponentially distributed with expectation \zeta, but it is not exactly true, they’re equal to 1+ an exponential rv with expectation \zeta -1: this implies that they are always larger than 1; please explain why (I guess you want M_A to be more resistant to B than S, but this is not straightforward for the reader and this should maybe be justified biologically?).
- Figure 1, please specify that the yellow and green lines are confounded in panels A and D (yellow is not visible). It is surprising that the yellow curve of optimal dosing is always so straight (there is no reason why it should be a line a priori) and even confounded with the green line in panel A: are you sure there is no mathematical result available when the drugs are not necessarily bacteriostatic? (see also comment by Reviewer 1 in 1st round)
- lines 295-304, can you be more specific about how you model cost? Otherwise, it is difficult to understand/believe your quite clear-cut conclusion that ‘too intermediate dosing strategies are sufficient to ensure P_E ≈ 1 even for very skewed mutation rates’.
- line 318, ‘This suggests that ignoring pharmacokinetics (as in the analytical solution) is not a fatal flaw’. Please reformulate.
- line 319, ‘Each replication event uses one arbitrary unit of resource, and the simulation begins with 10^9 units of resource, with a constant influx of 10^8 h−1. The maximum growth rate is now given by the Monod equation, with a resource affinity constant of 10^8’. Can you expand a little bit the paragraph starting here, explain your modeling assumptions, give symbols to these parameters (units, influx and affinity of resource), provide an equation for the effect on growth rate, explain your choice of parameter values and give an idea about how your results are robust or not to these choices? You say that ‘the basic relationship between mutation rate and optimal dosing concentrations persists’ but without a yellow curve, what we see is mainly the negative relationship between C_A/C_B and \mu_A/\mu_B, which is expected.
- line 333, ‘less beneficial’ => more hazardous/risky
- line 338 ‘but in several cases the dosing skew should never be raised above some maximum value.’ I must have missed something, can you specify which of your findings you are referring to here?
- line 351, ‘Our choices of functional forms for the drug-dependent mortality and replication rates in Equations 2 and 3 were crucial for the results that followed.’ Are you speaking of the mathematical results? Otherwise, it is a little worrying that these choices are crucial.
- line 366, ‘As a result, unlike with Bliss independence, skewed drug dosing ratios do not clear the infection slower.’ It is not clear to me how you conclude this from the previous considerations
- line 373, ‘in reality, there is no sharp cutoff’, please recall here why your assumption that the total drug dose is smaller than c can be seen as a cutoff
- line 408-409, if you want to interpret mutations in your model as HGT, you have to consider the rate of HGT within a host; under mass-action, the (per susceptible pathogenic bacterial cell) rate should be proportional to R, where R is the number of (commensal) bacteria carrying the resistance gene already present within the host, which itself depends in a nonlinear and time-dependent manner on the number, denoted I in this paragraph, of hosts ‘infected’ by this bacteria (see also comment by Reviewer 1 in 1st round). I understand that this rate is somewhat external to the dynamics you are studying, and does not interfere with it, but you should be a little more cautious when you say that it is approximately constant – it is at least time-dependent.
- line 414-415, ‘Thus, using a drug to which the commensal bacteria is susceptible could make resistance considerably less likely to evolve.’ You’re basically saying that due to possible HGT, it is better to use a drug for which there are no resistance genes already present in the microbial community. I’m not sure this is worth saying.
- Figures S3-S4: please say a word in main text or caption how to interpret the Z-shaped yellow curves. In Figures 3 and S2, you explain that beyond a certain threshold value of mutation rate ratio, the best strategy is to use only one drug. But what’s happening in Figures S3 and S4?
Please be so kind as to prepare a revised version of your manuscript addressing these points,
Best,
Amaury Lambert
Evaluation round #1
DOI or URL of the preprint: https://doi.org/10.1101/2024.05.04.592498
Version of the preprint: 1
Author's Reply, 22 Nov 2024
Decision by Amaury Lambert, posted 08 Sep 2024, validated 09 Sep 2024
Dear authors,
Let me first apologize for the delay in handing this decision - I have come through a very difficult summer at a personal level.
The two referees have written reports of high quality. They appreciated the manuscript but have a number of important comments that you are asked to address in your revision. A few points in particular have retained my attention:
- You have made a noticeable effort in relaxing some simplifying assumptions, except for one which seems particularly important. In the manuscript, the growth rates of bacterial populations are always constant - there is neither competition between populations nor time inhomogeneity due to drug elimination (see comments by Referee 1). Please assess the domain of validity of your results in relation to (at least one of) these assumptions. This point seems to be addressed in another preprint of yours cited in the manuscript. Since both manuscripts are rather short, you might consider merging them, as proposed by Referee 2.
- Please be more diligent in citing and comparing your work with existing literature on the topic of antibiotic resistance evolution (e.g., works cited by Referee 2). Also please provide more references from the "significant body of empirical literature" on combination therapy (line 28).
- You could easily address several comments of both referees, and at the same time improve clarity, by expanding a little bit your presentation of the model and of the consequences of your simplifying assumptions regarding parameter values :
- First, assuming that the beta's and the phi's are all equal to 1, G_S = g/((1+C_A)(1+C_B)). Then your assumption that S and M_B both have zero net growth rate when C_A = 0 and C_B = c implies g = \delta (1+c) and G_A = g/(1+C_B) (similarly with M_B). You should also say that under these assumptions, whenever C_A+C_B=c, G_S<D_S and G_A>D_A (similarly with M_B);
- Second, you should write the calculations that lead to (13) and emphasize in particular (see comment by Referee 1) the intermediate step showing that (up to the dividing factor 1+c) you have that \mu(1-P_D) = \mu_A C_A + \mu_B C_B and that (the expectation of) N_r = S_0/(C_A C_B).
Minor points:
- The division (or birth) rate of bacterial cells is called growth rate and denoted G. It is more common to define the growth rate as the difference between birth and death rate.
- Note that (11) can be obtained directly by saying that extinction does occur iff at each division event, we don't have both a mutation event and the survival of this mutant;
- Note that because you assume that the exponential decrease of S is deterministic, you have that: 1) N_r has a Poisson distribution with parameter given by the rhs of (8); 2) because N_r is a Poisson variable, equation (12) holds without approximation by taking the expectation of (11) (wrt the law of N_r), which results in (12) after replacing N_r with its expectation (i.e., the parameter of its law).
Please prepare a revised version of your manuscript addressing these points and and the points raised by the two referees.
Best,
Amaury Lambert
Reviewed by anonymous reviewer 1, 18 Jul 2024
Title and abstract
Does the title clearly reflect the content of the article? Yes
Does the abstract present the main findings of the study? Yes
Introduction
Are the research questions/hypotheses/predictions clearly presented? Yes
Does the introduction build on relevant research in the field? Yes
Materials and methods
Are the methods and analyses sufficiently detailed to allow replication by other researchers? Yes
Are the methods and statistical analyses appropriate and well described? Yes
Results
In the case of negative results, is there a statistical power analysis (or an adequate Bayesian analysis or equivalence testing)? Not applicable
Are the results described and interpreted correctly? Yes but interpretation could be improved
Discussion
Have the authors appropriately emphasized the strengths and limitations of their study/theory/methods/argument? Yes except for two key assumptions as detailed below.
Are the conclusions adequately supported by the results (without overstating the implications of the findings)? Yes but limited in scope.
Review of Delaney et al.:
In this paper, the authors investigate the probability of extinction of a bacterial population under multidrug antibiotic treatment. Specifically, they model the stochastic birth-death dynamics of bacterial cells, and the de novo evolution of resistance to drugs A or B that occurs with respective rates muA and muB. They investigate the concentrations CA, CB (with a constraint CA+CB = c) that maximize the probability of extinction. They find that in simplifying conditions, the ratio CA/CB is the square root of muB/muA. Indeed, drugs A and B have a similar effect on sensitive cells, and it is more efficient to apply a larger concentration of drug B if there are more A-resistant cells.
All in all, I think the paper is clear and presents an interesting model on an important problem. But I am not sure of the generality of the findings. First of all, the main result depends on somewhat hidden assumptions on the interaction between the two drugs for birth and death (defined in equation 2). Second, the assumption that there is no competitive release of the resistant strains is not discussed (mentioned line 68, "ignored resources constraints to growth"). That is, growth is not density limited and therefore does not depend on the total density of bacterial cells, which is expected to decline strongly in the presence of treatment. Competitive release is an important biological phenomenon and as such is most often incorporated in within-host model of AMR evolution. For example, competitive release can make resistance harder to evolve when drug dose is low, because sensitive cells are still at sufficiently high density to competitively inhibit the growth of resistant strains (see the papers by Troy Day, Andrew Read, and collaborators). Competitive release may thus change the results but this is not discussed.
For convenience, I list below the assumptions that are made in the paper and how they are relaxed (or not):
Assumptions in main model:
0) Implicit assumption on the interaction between drugs, embedded in the function forms defined in equation (2) -> not relaxed, briefly mentioned
1) absence of competitive release -> not relaxed, not discussed
2) the mutation rates mu_A and mu_B do NOT depend on antibiotic concentration -> relaxed by exploring distributed z value.
3) the efficacy and toxicity of both drugs are the same (lines 89-90) -> discussed around line 232
4) the "toxicity function" implies that total concentration is limited to exactly c (= sharp cutoff above which it is too toxic) (discussed lines 235)
Extra-simplifying assumptions for the simple analytical result:
5) Resistant strains are UNAFFECTED by arbitrarily high drug concentration(line 129-130) -> important, relaxed later
6) Shape parameters beta are unity -> relaxed
7) No cost of resistance -> relaxed
8) Bacteriostatic (not needed I think? See comment below)
Generally, I believe it would be useful to present the outcome a null model where the drugs don't interact by default for growth (as is the case currently), and in so doing relax assumption (0). Relaxing assumption (1), or at least some discussion of this assumption and how it limits applicability of the results, would also be necesary.
Another, linked general comment: the probability of extinction of the bacterial population depends solely on ratios D/G, as both the P_D and the N_r only depend on ratios D/G. Is there scope to use this analytical result to build a bit more intuition about the consequences of equation (12)? In particular, if drugs A and B do not interact (a distinct assumption than that of the authors), I expect N_r to be independent of the doses of C_A / C_B (only depend on total c) and therefore effects of C_A / C_B only emerge from impact on P_D. What happens in that case? Exploring this for generic forms of the (D/G)(C_A) function may be conceptually useful. In general, it would be helpful to delineate what in the result emerges from the P_D term, vs. the N_r term.
Comments:
---------
Methods:
--------
14: optimal, please define wrt what criterion
27 "synergistically" -> indeed I understand that there is some form of synergy in the model, please state so.
28 "Combination therapy is supported..." please specify in which type/context of infections, what pathogen.
Around line 33, could introduce here the caveat that in many cases, resistance is introduced not by de novo mutation, but acquired by transmission of a resistant strain, or evolved by horizontal gene transfer (the latter is discussed).
The authors might find this reference interesting: https://journals.plos.org/plosbiology/article?id=10.1371/journal.pbio.1002299
Line 45, the cdc webpage referenced for Mtb no longer exists as of 16th July.
Table 1: please specify the unit of mutation rate estimate (per generation? genome-wide?). To what antibiotic dose does it correspond?
Line 66, please clarify whether phi can vary continuously between 0 or 1, or if it is always exactly 0 or 1.
Equation (2): the implicit assumption of synergy between drugs built in equation (2) should be commented more. For example, with beta_j = 1, z_{i,j} = 1, the growth rate with C_A = 1 and C_B = 1 is equal to 25% of the growth rate without drug. The growth rate with C_A = 2 and C_B = 0 is equal to 33% of the growth rate without drug. Thus, there is a built-in assumption that the two drugs are not equivalent and that it is best to apply some of the two drugs (and not exclusively one drug). It would also probably be clearer to compare explicitly with a model without such assumption, that is one where applying C_A or C_B is equivalent in terms of effect on growth rate (e.g. G_i = g_i * exp(-(C_A + C_B))).
Line 75 I was surprised to read that the ODE model is actually never used. Would be clearer to define the model in terms of individual reactions (birth and death) and then explain how this is implemented. There is no need to formulate an ODE model at all, if it is not used.
Could specify below equation (4) that the mutation rates mu_A and mu_B do NOT depend on antibiotic concentrations, and this assumption is relaxed later on. Again this is a critical assumption, as resistance to high drug concentration is less frequent.
Line 87 I suspect the actual value of c is quite important, as this will determine the domain of non-linearity of the E function in which C_A + C_B is. Would be good to test several alternative values of c (e.g. lower / higher than 2)
Line 89-90, "drug concentrations are scaled to be in units standardised to the potency of the drug in question" -> this together with C_A + C_B determines toxicity, loses some generality, correct? That is, the efficacy and level of toxicity of both drugs are the same. Would be good to state this assumption explicitly here (it is discussed around line 232).
Line 94-96: I think this is the key assumption explaining the result: the shape of the E(.) function means it is not optimal to use only the less evolvable drug. But this is somewhat hidden at the end of the Method paragraph, would be good to place it more prominently when results are derived, and in the discussion.
Results:
--------
Equation 5: Would be nice to give an explanation of the mu_A / (mu_A + mu_B) terms.
Equation 11: Would be nice to give a bit of interpretation of this simple equation
Line 136-138: I do not understand the condition that when only drug A is applied (C_A = c, C_B = 0), then net growth rates of the susceptible and B-resistant strains are both zero: G_S - D_S = G_M_B - D_M_B = 0. If the antibiotic is bacteriostatic, then I expect G_S = 0 and G_M_B = 0 in these conditions, i.e. growth is totally inhibited. In which case G_S - D_S and G_M_B - D_M_B should be negative. Please clarify this condition in relation to the fact that the drugs are bacteriostatic.
Line 154-156: I don't understand why the result on bacteriocidic drugs is deprecated (e.g. 'bacteriostatic' is mentioned as an assumption line 135): if the result also holds mathematically for bacteriocidic (and even if intermediate steps are more complicated), this is a result just a strong as that for bacteriostatic.
Line 161: could explain briefly why value of P_E is lower for bacteriostatic (this build intuition for why optimal dosing strategy is biased towards the bacteriostatic drug).
Line 176: what is z_W? Is it xi?
Line 177 it was not fully clear why the optimal strategy tends to equal amounts of both drugs; if both drugs are ineffective, why would it matter to apply 50-50 of both drugs?
Line 214 again here, would be nice to write the intuition explicitly.
Line 260 I think it is incorrect that "the process of HGT would be equivalent to the process of mutation", since mutation depends on density S but HGT from another species does not.
Figure S5: it would be still nice to show the yellow theoretical line even if the theory is for constant drug concentration